Machine vision enabling the factories of the future
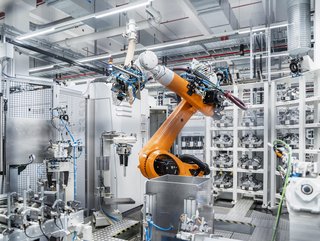
Think of a factory of the future and you might imagine sci-fi-like advanced technologies, with highly-automated robots.
But with Industry 4.0 technologies like AI, 5G and IoT, this future is closer than you might think.
Intel describes machine vision technology as giving industrial equipment the ability to “see” what it is doing and make rapid decisions based on what it sees.
One of the founding technologies of industrial automation, machine vision has helped improve product quality, speed production, and optimise manufacturing and logistics for decades. Now this proven technology is merging with artificial intelligence and leading the transition to Industry 4.0.
Central to those visions of the factory of the future are autonomous robots, capable of performing highly complex tasks with incredible accuracy. As Omkar Nisal, Managing Director UK & Ireland at Wipro Ltd explains, machine vision has played a key role in the evolution of industrial robotics.
“Machine vision has empowered industrial robots with the ability to see things with high resolution and speed. Earlier robots could only use sensors for measuring vibrations, acoustics, temperature and depth to monitor and feel surroundings.
“Now, the journey of automation that started from basic sensors and microcontrollers has now accelerated with the addition of machine vision. It has now evolved into a more complex hybrid AI-led machine which can make fast decisions from multiple types of connected devices like sensors and cameras.”
This evolution, Nisal adds, has been primarily triggered by advancements in technologies like AI and chip designs. “It has also enabled new industrial use cases which seemed impossible in the past; visual inspections of oil rigs, automated quality inspections and remote monitoring of safety-critical assets have become a new reality, which we at Wipro are driving for some of our key customers.”
How machine vision is driving automation innovations
From smart warehouses to subsea inspections, every new advancement in automation is driven by machine vision being at the forefront of such use cases.
“For example,” Nisal explains, “oil and gas explorations in the deep sea can reach great depths because of machine vision. A magnetic crawler attached with a camera can reach great heights and remote places in industrial plants and perform difficult inspections.
“A robot can detect internal cracks in a gas pipeline proactively, thereby saving millions of dollars. Robots can proactively alert operators by grading the corrosion of pipes from drone pictures and videos, helping operations be a lot more preventive while reducing systems downtime. This grading is done using complex machine vision and deep learning techniques.
“Overall, the relationship between industrial robotics and machine learning is becoming increasingly symbiotic, and this is expected to accelerate as technologies continue to advance.”
Central to the advances in machine vision are innovations in edge computing. Increasingly powerful edge computing, plus a growing universe of deep learning models for AI, are radically expanding what machine vision can do.
“Recent innovations in edge computing have tremendously helped accelerate the use of machine vision in manufacturing,” Nisal explains.
“The affordability of high compute devices has meant that heavy deep learning algorithms have now become a regular occurrence on many vision implementations. The advancement of technology from basic microcontrollers to sophisticated yet compact powerhouses has helped the manufacturing industry look at use cases like visual quality inspections, automatic part alignments and accurate packaging.
“This is helping the industry with reduced waste, improved quality, faster inspection cycles and increased production throughput.”
Deep learning supports and boosts machine vision
Deep learning-based algorithms for machine vision and predictive analysis have become a game changer for digital inspections. These AI algorithms have wide applicability, ranging from automated site inspections to quality analysis of materials and products for detecting cracks, anomalies, orientations, colour, and thickness.
“Deep learning has played a crucial role in enabling pre-trained models for object detection, people detection, segmentation, and classification,” Nisal comments. “It allows transfer learning for customising models for the industrial domain, which has resulted in faster turnaround times for machine vision.
“More recently, there are now optimised networks for edge devices, accelerating the adoption of machine vision.”
Private clouds are expected to play an important role in the future of industrial machine vision applications, with scalability and the ability to carry out detailed data analysis being just two examples of the benefits to be expected, Michel Spruijt, Chief Revenue Officer of Brain Corp told Technology Magazine recently.
“Autonomous mobile robotics are able to collect a tremendous amount of data, but customers expect that data to be parsed into digestible and actionable insights,” he explains. “The maturation of cloud technology will help expedite the process of pushing data from the edge to the cloud and then back down to the customer.”
With the future of industrial machine vision applications expected to reside in private clouds, cloud technology has an important role to play.
“Most of these vision solutions are built inherently on the cloud, as a result of initial cost advantages. Initially, deep learning algorithms demanded huge amounts of hardware,” Nisal comments.
“The earlier solution designs used cameras, sending images and videos to the cloud. This was advantageous for small use cases but, on a larger industrial scale, it soon became a problem. It caused network bandwidth issues due to thousands of images and videos being transferred onto the cloud which then started clogging the network.
“Going forward, the future of vision systems will be local image processing, either on the edge device itself or on private local cloud platforms. Having data processed locally solves many of the problems related to bandwidth, real time performance and data security.”