How to avoid AI pitfalls in business
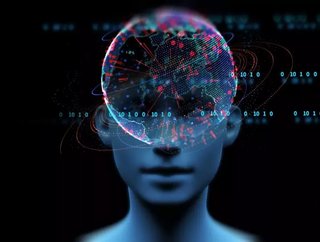
The use of AI in business is still in its infancy, but is already raising fundamental questions for businesses and their customers. While we’re figuring out answers, knowing how to avoid major AI business failure is arguably as important as knowing how to build great AI technology.
Many AI project pitfalls are technical in nature, and businesspeople have limited influence. But some relate directly to business aspects of AI work, and business stakeholders can make a big difference.
Below are five business-oriented challenges for AI work, and tips to avoid or handle them. They’re a result of anecdotal, personal and published experiences of AI projects that could have gone better.
Poorly Thought-Through Business Questions
An easy AI trap for the unwary is expecting AI to answer unreasonable or unrealistic business questions. This can lead to answers that are at best difficult to use constructively, or worse, meaningless or ambiguous.
For example if you have a customer retention problem, you might ask for AI that spots customers likely to leave. It seems sensible to assume that if you know who they are, you’re a step closer to doing something about it. The pitfall comes when you’re asked how accurately you want to identify them, because an obvious answer could be “as accurately as possible”, ideally 100%.
This is a problem if you’re in an industry where customers leave because of growing dissatisfaction over time (rather than individual triggers like price rises). That’s because the most accurate prediction of a decision to leave will be possible when a customer is most dissatisfied, typically just before they make the decision. By then, options to change their mind are likely to be fewer and harder than earlier in the relationship.
A better business question could have been asking AI to uncover early signs of dissatisfaction, ideally with some insight into the causes. With that kind of information, interventions are probably more likely to work.
A straightforward way to prevent this kind of problem is playing out how the AI results will be used in business terms. It’s also very good practice to extrapolate this a few quarters and years into the future, trying to visualise the longer term business impact of this and related AI solutions.
Unquantified or Unstated Business Goals
There’s plenty of material around describing how AI can benefit business, but much less on how to quantify that benefit. Without benchmarks and published data to help validate predicted returns on AI investments, business cases are likely to be riskier than other technology spend. But when everyone seems to be talking about and using AI, it can feel risky to not be doing AI work.
The pitfall for business leaders is starting AI work without clarity on what it’s expected to achieve, expressed in measurable terms. It may seem unlikely that businesses would do that, but anecdotal evidence suggests it’s surprisingly common. The reasons are complex, and the resolutions are challenging.
One possible solution is grouping AI spend with existing investments such as R&D. Another is setting “soft” business goals, consciously accepting latitude on results - including occasional failures. A third is using early projects to create your own baseline of metrics for future AI business cases.
Insufficient Focus on Business Impact
Like other IT work, AI business projects create technical outputs to help businesspeople solve business problems or improve business activities. And as with other IT work, solving problems or improving activities involves modifying or even transforming day-to-day work to make use of the technical outputs.
Today, good IT projects balance business and technology activities, but that’s not yet the norm in AI work. It’s not clear why, but it may be linked to AI being seen as a very specialist technical skill, and business people not being considered qualified to be involved.
At this early stage in the evolution of AI business work, one answer is to simply make sure business implementation is a standard part of any status reporting, including in particular for senior management. Another is to include business people in core AI teams, not just consult them occasionally for “user” input. This is part of a much bigger discussion, and we’re not even scratching the surface here.
Hiring the Wrong Skills
If you staff your AI projects in-house, you’ll need to hire AI skills such as data science. Alternatively, to use third parties for AI work, you’ll need a different profile of in-house AI skills e.g. to procure and manage data scientists or AI consultancies. A common pitfall here is hiring AI skills without being clear on what those should be and why.
It’s made harder by lack of consensus on how to structure AI teams or even where in an organisation they fit. Also, HR and procurement teams are rarely familiar with how to evaluate AI candidates or suppliers.
There’s no simple mitigation here either, but being aware should make it easier to avoid expensive mistakes, particularly around permanent hires. A useful rule of thumb might be to err on the side of flexibility until you have at least a clear AI sourcing strategy.
AI For the Sake of AI
The fifth AI pitfall is the easiest to spot and deal with. It’s the risk of falling into an “Emperor’s New Clothes” mindset, doing AI work primarily because of a desire to use AI, perhaps because everyone else seems to be. This goes hand-in-hand with insufficient thought on the kind of problem to solve with AI, and leads to projects lacking business focus, or trying to solve problems not suited to AI.
To avoid this, look for symptoms that a desire to use AI is driving a project, rather than a genuine, appropriate business problem.
Like surgery and soccer, success is the ultimate goal of AI projects, but avoiding failure can be as important - moreso sometimes. Avoiding these five and similar pitfalls puts you ahead of many others exploring AI in business.
By Was Rahman, CEO of AI Prescience and the author of AI and Machine Learning