AI is powering better recommendations on streaming services
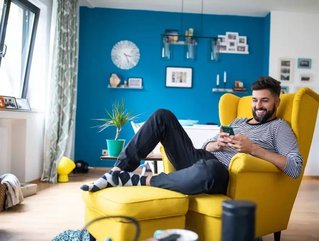
In 2021, for the first time in history, more people will pay for online streaming services than for traditional pay-TV. But the streaming market is now more crowded and competitive than ever before. In recent years, high-quality original programming has been the primary way streaming providers enhance and differentiate their services. Netflix is estimated to house around 1,500 TV series and 4,000 films, Amazon Prime Video is home to almost 20,000 titles, and a subscription to Disney+ adds around 7,000 more TV episodes and 500 films for viewers to choose from.
However, high-quality programming alone is not enough to keep consumers subscribed to a service. One of the most common problems today’s audiences face is finding something they want to watch. As recently as 2017, viewers were spending almost an hour a day searching for content. It is a daily dilemma which often results in endless scrolling before the consumer simply chooses something that vaguely interests them, because they do not want to waste more time searching for something truly compelling. The reality is that offering a superior user experience is the key to a video streaming provider breaking away from the competition and becoming the go-to service. The only way a streaming service can achieve this is by using AI and machine learning to gain a deep understanding of its content and serve more relevant and intuitive recommendations to audiences.
Currently, many streaming services are using content discovery systems which often provide simplistic and inaccurate recommendations. Many content discovery systems rely on basic metadata, which broadly labels content based on data points such as genre, the actors starring in it, or even just picking up on keywords in content titles. Think of it like this: how likely is it that after watching Marley & Me, the family comedy starring Owen Wilson and Jennifer Aniston, that the viewer will want to watch Marley, the biographical documentary on reggae icon Bob Marley?
The power of content
The output of recommendations will only be as good as the input. So when streaming platforms don’t know enough about their content, their recommendations will be poor. To take recommendation systems to the next level, streaming providers need to harness AI and machine learning technologies to gain a deep understanding of the content in a scalable way by analysing the audio and video file itself.
Content analysis based on AI and machine learning can have different neural networks to identify patterns in colour, audio, pace, stress levels, positive/negative emotions, camera movements and many other characteristics. It can then evaluate how similar each asset is to every other asset and combine this information with an AI engine that analyses a household’s watchlist, drawing together a more advanced and nuanced understanding of the content asset and its relevance at any particular time.
A user that watches a disturbing horror film on a Friday night may well want something more light-hearted immediately after, and a recommendation system that is being fed this type of detailed content data can offer this level of intuition. Over time, it can analyse each viewer’s consumption patterns and data points – not just each device, but each individual user profile – and perfectly tailor recommendations for their watch preferences, suggesting the right content, at the right time.
There’s a mood (category) for that
Understanding the content itself goes beyond just understanding similarity, it opens the door to a whole range of new use-cases that traditional metadata won’t allow you to tap into. With the emotional data of the content coming from the audio/video file itself, we can automatically curate entire mood categories and channels for viewers. One of the easiest ways streaming providers can reduce the amount of time viewers spend looking for content is to categorise by mood. The type of content we want to watch is often strongly related to how we feel at that particular moment, so grouping content by mood makes the user experience more intuitive. An advanced AI engine can analyse the intrinsic emotional profile of each content asset to create nuanced categories. For example, moods can be categorised as “tense, fast-paced horror” or “light-hearted escapism”. Therefore, someone who has just got home from work after a stressful day will know to avoid content in the first category if they want to watch something to unwind. Additionally, in group settings when there’s a lot of debate over what to watch, it’s much easier to find something that interests everyone by asking, “what is everyone in the mood for?” and then finding the appropriate category.
Right now, there’s a great number of streaming platforms available to consumers. Forward-thinking players that want to stand out from the crowd and build brand loyalty among consumers need to offer an enhanced user experience that is differentiated. The only way video streaming providers can achieve this is by using AI and machine learning to gain a deep understanding of their content, so they can better understand their customers and provide them with the best viewing experience possible.
By Marcus Bergström, CEO of Vionlabs