How Flex Logix designs chips for edge AI computing
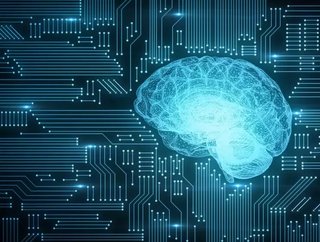
Historically, machine learning and neural networks have required centralised processing power, but as AI applications are becoming increasingly remote, such as in autonomous vehicles or the computer vision capabilities of a mobile phone, it’s vital that AI processing can be done in a more distributed manner.
In that vein, semiconductor firm Flex Logix Technologies designs chips and software for the acceleration of AI at the edge.
AI edge inference accelerator
The Mountain View, California-based company is currently producing chips and boards called InferX X1 which include features such as embedded Field Programmable Grid Arrays (eFPGA), which can add AI programming capabilities, and AI inference, which puts deep learning to use, in order to accelerate AI computing at the edge.
The company’s AI inference architecture is optimised for computer vision applications, with neural networks supported by tensor processors that rapidly connect compute and memory.
$55mn backing
Since the company’s foundation in 2014 it has raised nearly $70mn, with its latest Series D, announced yesterday, being by far its biggest to date - clocking in at $55mn. The round was led by Mithril Capital Management, alongside existing investors Lux Capital, Eclipse Ventures and the Tate Family Trust.
"We are impressed with the very high inference-throughput/$ architecture that Flex Logix has developed based on unique intellectual property that gives it a sustainable competitive advantage in a very high growth market," said Ajay Royan, managing general partner and founder of Mithril Capital Management. "This technology advantage positions Flex Logix for rapid growth in edge enterprise inference in applications such as medical, retail, industrial, robotics and more.”
Geoff Tate, CEO and Co-founder of Flex Logix said: "Our InferX X1 chips and boards will be available for mass production in mid 2021 along with availability of our InferX Inference compiler, which takes in Tensorflow Lite and ONNX neural network models and generates the code to run InferX X1 without the detailed programming other solutions require."