Large language models a welcome “wild west” for economists
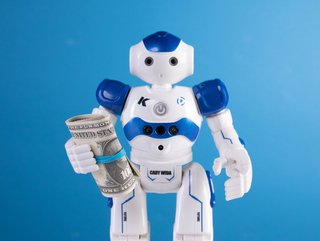
Economy experts believe large language models (LLMs) such as OpenAI’s ChatGPT can provide economists with new insights and should be welcomed for their creativity and opportunities for experimentation.
“I believe there is much that economists can learn from LLMs, not by chatting with the LLMs, but by deconstructing how they work,” says César A. Hidalgo
Director, Centre for Collective Learning, Artificial and Natural Intelligence Institute (ANITI), University of Toulouse and Corvinus Institute of Advanced Studies (CIAS), Corvinus University. “After all, LLMs are built on mathematical concepts that are powerful enough to help us simulate language. Maybe, understanding how these models work can become a new source of inspiration for economists.”
Hidalgo explains these models work by starting with a basic language-generating model that involves counting the number of times a word is followed by another in a large corpus of text. These sequences are called bigrams or 2-grams. While this method may not generate coherent text, it can still recognize that adjectives typically precede nouns in the English language, making "brown dog" a more common bigram than "dog brown."
LLMs, on the other hand, utilise n-grams to predict the probability of a word based on the previous words or tokens. However, as the number of words and n-grams increases, the matrices can become massive. For instance, a 10,000-word corpus can generate 100 million 2-grams, a trillion 3-grams, and 1,072 combinations for 18 grams, more than the amount of information that can be stored by every atom on Earth.
To address this issue, LLMs use neural networks to estimate a function that describes all these word sequences with just a few parameters. Although LLMs may have nearly a trillion parameters, they are still considered tiny compared to the infinite number of n-grams in Borges' library. These findings may aid in developing more effective LLMs for various natural language processing tasks.
“The result is models that begin to mimic knowledge. LLMs 'know' that tea and coffee are similar because they have learned that these words are used near words such as hot, drink and breakfast,” says Hidalgo. “By representing words, not as isolated entities but as nodes in networks, these models create the representations needed to generate language.”
Complex economy requires nuanced approach
Similar to the complexity in text where several words interact, economies also involve intricate interactions among various people and objects. While it's possible to categorise them into predefined groups such as capital and labour or activities such as agriculture, service, and manufacturing, these categorisations are inadequate representations of the economy's complexity.
Just like language models based on the idea of nouns, verbs, and grammar are incomplete models of language, models based on broad categorisations of economic activities are insufficient. The economy's complexity requires a more nuanced approach that captures the interactions between various factors rather than just dividing them into predefined categories.
“What LLMs teach us, is that there is a limit to our ability to capture the nuance of the world using predefined categories and deductive logic,” says Hidalgo. “If we want to get into the nitty-gritty, we need a mathematical toolbox that can help us capture systems at a finer resolution.
The concept of using network representations to understand complex interactions in economics is not a new one. In fact, there are branches of economics that have been using these ideas for a long time. Six years before the famous word embedding algorithm Word2vec was published, Hidalgo, together with three other colleagues, published a network representation of international trade. This network is technically a 2-gram that represents products based on their relationships with other products.
Like the coffee and tea example, this network recognises that drilling machines and cutting blades are related because they tend to be exported with similar sets of other products. It also distinguishes between tropical and temperate agriculture and manufactures t-shirts and LCD screens.
Over the last fifteen years, these methods have gained popularity among young economists and experienced practitioners. They provide the necessary tools to apply policy prediction ideas and anticipate the entry and exit of economies into different products and markets, which can aid economic development.
These methods have also resulted in "embeddings" for economics, such as the Economic Complexity Index. This metric is derived from a similarity matrix among economies and explains regional and international variations in long-term economic growth, income inequality, and emissions. These embeddings are vector representations similar to those used to describe a word in a deep-learning model.
“The ability of machine learning to gather, structure and represent data is creating opportunities for researchers across the board,” says Hidalgo. “From computational biologists looking to understand and predict the behaviour of proteins, to economic and international development experts looking to understand and predict the evolution of economies.
“Economists and computer scientists alike should welcome this new methodological revolution. It is a new wild west for creativity and experimentation.”
- Clever coders lead the way as Microsoft launches 365 CopilotAI Applications
- GPT-4 is live, with Morgan Stanley and Stripe early adoptersAI Applications
- Scientists reflect on the Harry Potter nature of AI chatbotsAI Applications
- GPT-3 language model matches humans in psychological testsAI Applications