Experts call on AI support for latest cybersecurity battles
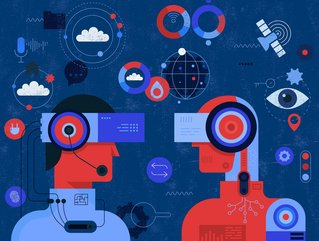
US researchers say deep reinforcement learning can offer a way for artificial intelligence to help protect computer networks in a world where state-sponsored hacking groups rub shoulders on the Dark Web with more traditional black hat types.
But the researchers say cybersecurity staff can relax, and even though businesses worldwide are reporting increases in ransomware, they’re not about to be replaced by an AI-powered workforce. For now.
Researchers with the Department of Energy's Pacific Northwest National Laboratory developed a simulation environment to test multistage attack scenarios involving different types of adversaries. This dynamic attack-defence simulation environment allowed them to compare the effectiveness of different AI-based defensive methods under controlled test settings.
According to their research, deep reinforcement learning (DRL) was effective at stopping adversaries from reaching their goals up to 95% of the time in simulations of sophisticated cyberattacks.
While other forms of artificial intelligence are standard for detecting intrusions or filtering spam messages, deep reinforcement learning expands defenders' abilities to orchestrate sequential decision-making plans in their daily face-off with adversaries. Deep reinforcement learning offers smarter cybersecurity by detecting changes in the cyber landscape earlier and the opportunity to take preemptive steps to scuttle a cyberattack.
“An effective AI agent for cybersecurity needs to sense, perceive, act and adapt, based on the information it can gather and on the results of decisions that it enacts,” says Samrat Chatterjee, a data scientist who presented the team’s work. “Deep reinforcement learning holds great potential in this space, where the number of system states and action choices can be large.”
The outcome of this research offers promise for a role for autonomous AI in proactive cyber defence. The development of such a simulation environment for experimentation is itself a win, as it offers researchers a way to compare the effectiveness of different AI-based defensive methods under controlled test settings.
By creating a dynamic attack-defence simulation environment, they could test multistage attack scenarios involving different adversaries, allowing them to compare the effectiveness of different AI-based defensive methods.
DRL helps respond to cyberattacks
Deep reinforcement learning (DRL) is emerging as a game-changing decision-support tool for cybersecurity experts. Unlike other forms of AI, which are limited to detecting intrusions or filtering spam messages, DRL allows defenders to learn, adapt, and make autonomous decisions in the face of rapidly changing circumstances. By orchestrating sequential decision-making plans, defenders can quickly respond to cyberattacks and prevent them from doing any damage.
One of the key benefits of DRL is its ability to detect changes in the cyber landscape early, allowing defenders to take preemptive steps to stop cyberattacks before they happen. With the threat of cyberattacks only set to increase, DRL offers a smarter and more proactive way to keep our computer networks safe.
The research findings were documented in a research paper and presented at a workshop on AI for Cybersecurity during the annual meeting of the Association for the Advancement of Artificial Intelligence in Washington, D.C. The development of DRL for cybersecurity defence represents an exciting step forward in the battle against cyber threats. As technology advances, researchers will undoubtedly discover new and innovative ways to harness AI for cybersecurity, ensuring that our systems remain safe and secure.
In addition to Chatterjee and Bhattacharya, authors of the AAAI workshop paper include Mahantesh Halappanavar of PNNL and Ashutosh Dutta, a former PNNL scientist.
Good decisions get a positive reward
DRL is a powerful decision-making tool that combines reinforcement learning and deep learning to excel in complex environments that require a series of decisions. Positive rewards are given to reinforce good decisions that lead to desirable outcomes, while negative costs discourage bad choices that result in unfavourable results.
This learning process through positive and negative reinforcement is similar to how humans learn many tasks. For instance, when a child completes their chores, they might receive positive reinforcement, such as a playdate with friends. Not doing their work could lead to negative reinforcement, such as losing digital device privileges. By mimicking this natural process of learning, DRL provides a promising approach to decision-making in the field of cybersecurity, enabling defenders to quickly adapt to changing situations and respond with greater efficiency.
“It’s the same concept in reinforcement learning,” says Chatterjee. “The agent can choose from a set of actions. With each action comes feedback, good or bad, that becomes part of its memory. There’s an interplay between exploring new opportunities and exploiting past experiences. The goal is to create an agent that learns to make good decisions.”
To evaluate the efficacy of four deep DRL algorithms, the team leveraged Open AI Gym, an open-source software toolkit, as a foundation for creating a custom and controlled simulation environment.
The researchers incorporated the MITRE ATT&CK framework, developed by MITRE, and included seven tactics and 15 techniques used by three separate adversaries. Defenders were given 23 mitigation actions to halt or prevent an attack from progressing.
The attack was divided into several stages, including reconnaissance, execution, persistence, defence evasion, command and control, collection, and exfiltration, when data is transferred out of the system. The adversary was declared the winner if they successfully reached the final exfiltration stage.
By testing these DRL algorithms under these controlled conditions, the team was able to assess the strengths and weaknesses of each approach, providing valuable insights into the potential of this technology to enhance cybersecurity defence strategies.
“Our algorithms operate in a competitive environment—a contest with an adversary intent on breaching the system,” says Chatterjee. “It’s a multistage attack, where the adversary can pursue multiple attack paths that can change over time as they try to go from reconnaissance to exploitation. Our challenge is to show how defences based on deep reinforcement learning can stop such an attack.”
“Our goal is to create an autonomous defence agent that can learn the most likely next step of an adversary, plan for it, and then respond in the best way to protect the system,” says Chatterjee.
Despite the progress, no one is ready to entrust cyber defence entirely to an AI system. Instead, a DRL-based cybersecurity system would need to work in concert with humans, says coauthor Arnab Bhattacharya, formerly of PNNL.
“AI can be good at defending against a specific strategy but isn’t as good at understanding all the approaches an adversary might take,” says Bhattacharya. “We are nowhere near the stage where AI can replace human cyber analysts. Human feedback and guidance are important.”