HPE: Businesses Face Overconfidence Trap in AI Strategy
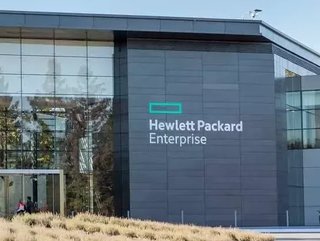
Artificial intelligence (AI) has captured the imagination of businesses across industries, promising transformative efficiencies, innovative solutions and competitive advantages. However, as organisations rush to embrace this game-changing technology, a sobering reality emerges: many are falling into an AI overconfidence trap, overlooking critical blind spots that could undermine their AI strategies and investments.
A recent global report commissioned by Hewlett Packard Enterprise (HPE) unveils a concerning disconnect between businesses' confidence in their AI readiness and the actual gaps in their approaches. The study, which surveyed over 2,000 IT leaders from 14 countries, found that nearly half (44%) of respondents believe their organisations are fully set up to realise the benefits of AI. Yet, the findings reveal significant deficiencies that could impede successful AI transformations.
“There’s no doubt AI adoption is picking up pace, with nearly all IT leaders planning to increase their AI spend over the next 12 months,” said Sylvia Hooks, VP, HPE Aruba Networking. “These findings clearly demonstrate the appetite for AI, but they also highlight very real blind spots that could see progress stagnate if a more holistic approach is not followed. Misalignment on strategy and department involvement – for example – can impede organisations from leveraging critical areas of expertise, making effective and efficient decisions, and ensuring a holistic AI roadmap benefits all areas of the business congruently.”
Data: The fuel for AI success
Strong AI performance hinges on the quality of the data inputs, a fact that organisations acknowledge by labelling data management as one of the most critical elements for AI success. However, the report exposes alarmingly low data maturity levels. Only 7% of organisations can run real-time data pushes and pulls, enabling innovation and external data monetization. Just 26% have established data governance models and can run advanced analytics.
Fewer than six in 10 respondents said their organisation is completely capable of handling key stages of data preparation for AI models, such as accessing (59%), storing (57%), processing (55%) and recovering (51%) data. This discrepancy not only risks slowing down the AI model creation process but also increases the probability of inaccurate insights and a negative return on investment (ROI).
While confidence levels appear high regarding network infrastructure and compute capacity to support AI traffic and workloads, a concerning knowledge gap emerges. Less than half of IT leaders admitted to having a full understanding of the demands of various AI workloads across training, tuning, and inferencing. This lack of understanding raises serious questions about organisations' ability to accurately provision the necessary resources, potentially leading to performance bottlenecks, inefficiencies and suboptimal outcomes.
The report reveals fragmentation in organisations' AI strategies. Over a quarter (28%) of IT leaders described their organisation's overall AI approach as "fragmented," with 35% creating separate AI strategies for individual functions and 32% setting different goals altogether. This siloed approach not only hinders cross-functional collaboration and synergies but also risks duplicating efforts and misaligning priorities.
Perhaps even more alarming is the apparent disregard for ethics and compliance considerations. Legal/compliance (13%) and ethics (11%) were deemed the least critical for AI success by IT leaders. Shockingly, almost one in four organisations (22%) are not involving legal teams in their AI strategy conversations.
The risks of overconfidence
The consequences of overlooking these blind spots are far-reaching and potentially damaging. Businesses lacking a robust AI ethics policy risk developing models that lack proper compliance and diversity standards, resulting in negative impacts on brand reputation, loss of sales, costly fines, and legal battles.
Moreover, the quality of AI model outcomes is directly tied to the quality of the data they ingest. With data maturity levels remaining low and half of IT leaders admitting to a lack of full understanding of IT infrastructure demands across the AI lifecycle, the risk of developing ineffective models, including the impact of AI hallucinations, increases significantly. Additionally, the enormous power demands of AI models can contribute to unnecessary increases in data centre carbon emissions, further eroding the ROI from capital investments in AI and negatively impacting a company's brand.
As organisations navigate the world of AI, it is crucial to adopt a holistic and carefully considered approach. From training and tuning models on-premises, in a colocation, or in the public cloud, to inferencing at the edge, AI has the potential to transform data into actionable insights across every device on the network.
However, businesses must carefully balance the desire to be an early mover with the risk of not fully understanding the gaps across the AI lifecycle. Failing to do so can result in large capital investments delivering a negative ROI, undermining the very advantages AI promises.
The path to AI success lies in acknowledging and addressing these blind spots. Organisations must prioritise data maturity, aligning data management practices with their AI ambitions. They must also develop a comprehensive understanding of the compute and networking demands across the entire AI lifecycle, ensuring appropriate resource provisioning and infrastructure scalability.
“AI is the most data and power intensive workload of our time, and to effectively deliver on the promise of Gen AI, solutions must be hybrid by design and built with a modern AI architecture,” said Dr Eng Lim Goh, SVP for Data & AI at HPE. “From training and tuning models on-premises, in a colocation or in the public cloud, to inferencing at the edge, Gen AI has the potential to turn data into insights from every device on the network. However, businesses must carefully weigh the balance of being a first mover, and the risk of not fully understanding the gaps across the AI lifecycle, otherwise the large capital investments can end up delivering a negative ROI.”
******
Make sure you check out the latest edition of AI Magazine and also sign up to our global conference series - Tech & AI LIVE 2024
******
AI Magazine is a BizClik brand