The dynamic between humans & AI in tackling financial crime
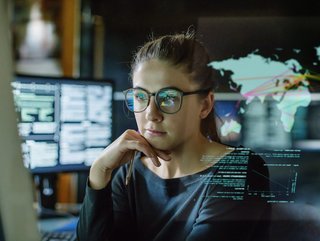
Conversations about AI have often revolved around its role in replacing humans. This is understandable given the lightning speed at which AI is advancing, particularly with the emergence of technologies such as ChatGPT. With that said, the fight against financial crime isn't a zero-sum game – it's a balancing act between the analytical prowess of AI and the nuanced understanding of humans. Therefore, the approach between humans and AI must be a collaborative one.
This article aims to shed light on the necessities and complexities of human-AI collaboration against financial crime. We'll delve into the distinct roles both parties play; from AI's relentless efficiency in detecting unusual patterns to the human knack for contextual judgement. We'll explore the feedback loop between human decisions and AI learning, the challenges to maintain a balanced partnership, and the potential evolution of this dynamic with technologies such as generative AI.
Understanding the roles of humans and AI in financial crime
In the complex world of financial crime, AI, and data analytics more broadly, have become invaluable tools.
With the rise of digital banking and online transactions, the sheer volume of data makes it nearly impossible for human analysts to thoroughly review and detect suspicious activity. This leaves room for potential crimes to go undetected, putting both banks and its customers at risk. Fortunately, through machine learning algorithms and advanced analytics, AI can quickly and accurately analyse large amounts of data, identifying patterns and anomalies that indicate fraudulent activity that a human might have otherwise missed. This not only helps prevent financial crimes but also saves time and resources for financial institutions.
Investigations, however, are not a solo act. Humans play a crucial role in reviewing these AI-detected cases, making critical decisions based on AI recommendations. This symbiotic arrangement creates a more efficient and effective approach in combating financial crime. For instance, an AI system may flag a potential fraudulent transaction, but a human is needed to review and ultimately decide to accept or reject it. This human-in-the-loop approach can help minimise false positives and ensure a balanced system.
Despite the pattern recognition capabilities of AI, a wider understanding of the world and its current affairs is crucial. Financial crime is not just about numbers and data, but also involves complex reasoning and nuance in decision making. Humans are able to consider various factors and make judgments based on their knowledge and experience, which AI may not be able to do. Additionally, AI operates solely on the data it has been trained on, which can sometimes be incomplete or biased. This highlights the importance of human involvement, as they can provide a critical perspective that AI may not be able to capture.
Impact of human decisions on AI recommendations
The importance of human involvement in investigations cannot be understated, however, this is not without its pitfalls. Supervised machine learning models are commonly used in fraud and money laundering detection. Using human labelled or annotated historical data, such algorithms learn to associate attributes of transactions with investigator decisions to identify activities that may be deemed suspicious.
Supervised algorithms therefore operate as part of a feedback loop. One might liken it to a dance, the AI makes a move, the investigator responds, and the AI adjusts its next move based on that response. However, there exists the potential for bias or error in human decision-making, which could unwittingly skew AI recommendations. Human decisions, such as accepting or rejecting transactions, leave indelible marks on how an AI learns.
One solution for minimising model bias is by adopting a combination of supervised and unsupervised techniques. Unsupervised learning is designed to find unusual hidden patterns in unlabelled data. This can be a more objective and unbiased approach. By utilising a variety of detection techniques, investigators can gain a more holistic perspective of activities being monitored.
In addition, the spotlight shines on the critical importance of continuous monitoring and evaluation. This ensures that the AI system’s recommendations retain their accuracy and fairness, safeguarding against any inadvertent skewing outcomes resulting from human decisions.
Large Language Models - A new dynamic
The advent of Generative AI, particularly large language models (LLMs), is set to revolutionize the way investigators interact with AI.
OpenAI's ChatGPT, among other tools, is currently being utilised in various fields for different purposes. It is only a matter of time before it becomes widely used in the financial crime industry as well.
Two key capabilities of LLMs stand out as potential game-changers:
- Handling Unstructured Data: LLMs are trained on a huge corpus of unstructured data allowing it to easily decipher information from data in various forms.
- Advanced Reasoning: LLMs can intelligently perform complex tasks based on user requests.
LLMs unlock the ability to automate tasks that were once thought to be too complex for AI. This includes querying databases, extracting information from documents, interpreting, aggregating, and contextualising data.
This takes assisting investigators to a new level by providing real-time information and analysis of data from different sources. For example, if a suspicious transaction is flagged, an AI chatbot can quickly gather relevant data from databases, AI models, and documents and present it to the investigator in a summarised, focused format, allowing them to make informed decisions in a timely manner.
It is easy to envision a future where investigations evolve into a conversation between AI and humans. The AI acts as an assistant gathering key information to the investigator, who ultimately makes the final decision.
Conclusion
In the intricate dance of combatting financial crime, both humans and AI systems must be synchronized. This article has highlighted the importance of understanding the dynamic interplay between humans and AI, the importance of human decision-making in shaping AI recommendations, and the need for continuous monitoring and evaluation of these systems. Despite potential challenges such as bias, the benefits of a collaborative approach are unequivocal.